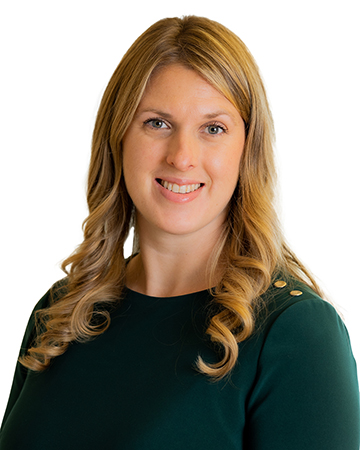
Artificial Intelligence: Legal and Regulatory Issues for Financial Institutions
Over the last six months, artificial intelligence (AI) has captured the public imagination in a way it never has before. A new generation of AI-powered language models make use of a deep learning architecture known as a transformer. Through the transformer architecture, AI can generate coherent and contextually relevant text based on input prompts provided by users. Trained on an extensive dataset, such AI predicts the next word in a sequence of text, allowing it to produce human-like written content. Its natural language processing capabilities have allowed it to be applied in various fields, ranging from content generation to translation and summarization.
AI combines computer science and structured data sets to create programs that perform tasks which typically require human intelligence, such as reasoning, learning and decision-making. “Real” AI traditionally refers to AI systems that attempt to demonstrate a broad range of cognitive abilities that may be perceived as being similar to those of a human being. Although large language model (LLM)-based AI software have demonstrated the ability to produce coherent and contextually relevant text based on input prompts, it is crucial to recognize that such AI software are not “real” AI in the traditional sense. Rather, these are powerful tools that excel at predicting statistically the next word in a given sequence.1 In fact, many LLM-based AI software lack the ability to truly comprehend or reason beyond the patterns they observe in the text, and cannot form independent thoughts, reason through complex problems or make decisions based on abstract concepts.
Footnotes
1) Scott W. Bauguess, Acting Director and Acting Chief Economist, DERA, SEC, “The Role of Big Data, Machine Learning, and AI in Assessing Risks: a Regulatory Perspective,” Champagne Keynote Address (June 21, 2017), https://www.sec.gov/news/speech/bauguess-big-data-ai (noting that latent dirichlet allocation “measures the probability of words within documents and across documents”).